Introduction
Gone are the days when cloud computing was simply a way to store data or run applications remotely. Now, cloud platforms function as intelligent ecosystems capable of learning, predicting, and self-optimizing due to AI and ML technologies. For businesses, this shift isn’t about improving system efficiency but unlocking new possibilities and freeing up human talent for strategic, high-impact tasks.
AI and ML-powered cloud automation enables businesses to anticipate disruptions before they occur, automate tedious processes that once consumed countless hours and transform raw data into actionable insights in real-time. This blog explains how AI and ML are elevating cloud automation beyond simple task execution, making the technology a strategic pillar for growth, innovation, and long-term sustainability.
What is Cloud Automation?
Cloud automation is the process of using specialized software tools and technologies to automatically manage, configure, and control cloud engineering services and solutions without the need for constant human oversight. Instead of manually handling tasks like server provisioning, application deployment, resource scaling, and security patching, businesses can automate these processes to run seamlessly in the background.
It reduces human errors and speeds up operations while ensuring systems can scale on demand and maintain consistent performance even during unexpected spikes in usage. When businesses integrate AI and machine learning into cloud automation, the systems become even smarter, using data insights to predict needs, self-correct issues, and optimize resource allocation in real time.
Why AI and ML are the Driving Forces Behind Next-Gen Cloud Automation?
Unlike traditional automation, which relies on static scripts and predefined rules, AI and ML enable cloud systems to learn from patterns, predict future demands, and make real-time decisions without manual input. Their role goes far beyond task automation as they bring real-time adaptability, predictive insights, etc., addressing the most critical pain points businesses face as they scale.
AI and ML improve resource allocation by analyzing workload patterns and automatically adjusting cloud infrastructure to match demand. This ensures businesses never overpay for idle resources or face downtime from sudden traffic surges, optimizing both cost and performance without human intervention.
These technologies enable proactive defence mechanisms. AI models can analyze vast streams of network data to identify vulnerabilities or detect abnormal activity in real-time, reducing the risk of breaches and strengthening cloud security with continuous learning and self-updating policies.
Moreover artificial intelligence and machine learning upkeep a reality. Rather than waiting for systems to fail, cloud automation platforms embedded with machine learning can flag potential performance issues, hardware faults, or software bugs before they happen, drastically reducing downtime and preventing costly disruptions.
Along with that, AI and ML technologies allow cloud systems to scale dynamically and intelligently. Based on real-time data, cloud platforms can allocate computing power, storage, and networking resources to meet fluctuating business demands automatically — something human-managed systems struggle to do efficiently at scale.
Business Use Cases for AI and ML in Cloud Automation
Businesses are leveraging AI ML development services to automate, optimize, and scale their cloud-based operations. By embedding intelligence into cloud automation, organizations across industries are transforming reactive workflows into proactive, self-optimizing systems. Here’s how different sectors are putting AI and ML-powered cloud automation to work.
Cloud Automation in Healthcare
In the healthcare industry, cloud automation integrated with AI and ML is helping organizations manage vast amounts of patient data, streamline operations, and improve care delivery. Machine learning models can automate the classification and storage of medical records while AI-driven systems handle predictive resource allocation.
Cloud Automation in Finance
Financial institutions rely on cloud automation to manage high-frequency transactions, fraud detection, and data compliance at scale. AI and ML algorithms automate fraud monitoring by learning patterns of legitimate vs. suspicious behavior and flagging anomalies in real time. Moreover, cloud environments also auto-scale during peak trading hours or financial reporting periods.
Cloud Automation in Retail & Ecommerce
AI and ML-powered cloud automation dynamically manages recommendation engines, adjusts server loads for seasonal spikes and automatically scales cloud infrastructure based on traffic, providing personalized customer experiences. Inventory management and order fulfilment systems also use AI to forecast demand and optimize stock levels across warehouses.
Cloud Automation in Manufacturing
In manufacturing, cloud automation with AI and ML helps businesses manage supply chains, monitor equipment health, and streamline production workflows. Predictive maintenance algorithms detect when machines are likely to fail and trigger automatic system alerts or maintenance schedules. AI-powered systems can also balance workloads across cloud-connected production sites to ensure efficiency and prevent downtime.
Cloud Automation in Logistics & Transportation
AI and ML models optimize delivery routes, predict shipment delays, and manage fleet resources through cloud-based platforms. Automated systems can adjust resource allocation during peak shipping seasons, helping companies maintain service quality while controlling costs.
Conclusion
The future isn’t about choosing between human capability and automation; it’s about building systems where both work in sync. AI and ML are setting the stage for businesses to move from managing processes to orchestrating possibilities — and cloud automation is the platform making it happen. The real transformation lies not in adopting new tools, but in adopting new thinking: letting data drive decisions, letting intelligence guide scale, and letting automation reshape how businesses respond to change.
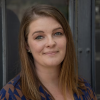
Lynn Martelli is an editor at Readability. She received her MFA in Creative Writing from Antioch University and has worked as an editor for over 10 years. Lynn has edited a wide variety of books, including fiction, non-fiction, memoirs, and more. In her free time, Lynn enjoys reading, writing, and spending time with her family and friends.