Generative AI is currently the talk of the tech world, and for good reason. Its capability to create content, mimic human-like interactions, and innovate across various domains has made it a game-changer for businesses. The numbers speak volumes about its rising prominence. The global market size for generative AI was valued at $13.24 billion in 2023 and is projected to skyrocket to $112.47 billion by 2032, growing at a compound annual growth rate (CAGR) of approximately 26.83% from 2024 to 2032. This explosive growth shows the immense potential and widespread adoption of generative AI technologies. At the center of generative AI lies data—the fundamental element that fuels its capabilities. Without vast and diverse datasets, generative AI would lack the foundation needed to learn, adapt, and produce innovative outputs. In this blog, we will delve into the critical importance of data in generative AI.
Importance of Data in Generative AI
The significance of data in generative AI cannot be overstated. Data is the lifeblood that powers generative models, enabling them to learn, generate, and innovate. Here are key aspects that highlight the critical importance of data in generative AI:
1. Fuel for Training Models
Data is the essential fuel for training generative AI models. These models, whether neural networks, transformers, or other advanced architectures, rely heavily on vast amounts of data to learn patterns, structures, and relationships. Without diverse and comprehensive datasets, these models would struggle to produce accurate and meaningful outputs. High-quality training data allows AI systems to understand nuances and complexities within the data, leading to more sophisticated and human-like generative capabilities. This foundational role of data ensures that the AI seamlessly performs tasks and adapts and improves over time.
2. Enhancing Model Accuracy
The accuracy of generative AI models is directly correlated with the quality of data they are trained on. High-quality data, characterized by its accuracy, completeness, and relevance, ensures that generative AI models can produce precise and reliable outputs. When trained on clean, well-structured data, models are less likely to generate errors or produce biased results. This is particularly crucial in applications where accuracy is paramount, such as medical diagnostics, financial forecasting, and autonomous driving. By ensuring that the data fed into the models is of the highest quality, developers can significantly enhance the performance and trustworthiness of generative AI systems.
3. Diversity and Robustness
Diverse datasets contribute to the enhancement of generative AI models. When models are exposed to a wide range of data types, sources, and variations, they become more versatile and capable of handling different scenarios and inputs. This diversity helps in building models that are more resilient to anomalies and better at generalizing from the training data to new, unseen situations. For example, exposure to different dialects, languages, and contexts in natural language processing ensures that AI can understand and generate applicable text across various user groups and applications. Diversity in data thus directly contributes to the creation of robust and adaptable generative AI systems.
4. Data-Driven Innovation
Data is the cornerstone of innovation in generative AI. By analyzing vast datasets, AI models can uncover hidden patterns and insights that might not be apparent to human researchers. This capability enables the development of new applications and solutions across various industries. For instance, in pharmaceuticals, generative AI can analyze molecular data to propose novel drug candidates. Similarly, new art forms or music compositions can be generated in the creative industry by learning from extensive datasets of existing works. This data-driven approach to innovation allows generative AI to push the boundaries of what is possible, leading to groundbreaking advancements.
5. Personalization and User Experience
Generative AI leverages data to create highly personalized user experiences. By analyzing user data, such as preferences, behaviors, and interactions, AI models can generate content tailored to individual needs. This personalization enhances user satisfaction and engagement, making applications more intuitive and user-friendly. For example, in e-commerce, generative AI can create personalized product recommendations, while entertainment can generate customized playlists or content suggestions. The ability to provide personalized experiences is a key competitive advantage, driven by the effective use of data in training and refining AI models.
6. Continuous Improvement and Learning
Data plays a crucial role in continuous improvement and learning process of generative AI models. They can adapt to changing environments and evolving user needs by constantly feeding new data into the models. This continuous learning process ensures that generative AI systems remain relevant and effective over time. For example, generative AI chatbots can improve their responses to customer service by learning from new customer interactions. AI models can update their diagnostic capabilities in healthcare by incorporating the latest medical research and patient data. Continuous improvement through data enhances the performance of generative AI and further extends its applicability to a wider range of dynamic scenarios.
Future Trends in Data for Generative AI
Generative AI is poised to evolve rapidly, driven by data collection, processing, and management advancements. Here are some future trends that are likely to shape the landscape of data in generative AI:
1. Advances in Data Collection and Analysis
The methods and technologies for data collection and analysis are expected to undergo significant transformations. Emerging technologies like edge computing and IoT will enable real-time data collection from many sources, enhancing the detail and immediacy of data available to AI models. Additionally, advancements in data analysis tools, incorporating AI and machine learning techniques, will allow for more sophisticated and nuanced insights from the collected data. This will empower generative AI models to become more accurate and context-aware, driving better outcomes across various applications.
2. Integration of Synthetic Data
The use of synthetic data is expected to rise as it provides a solution to the challenges of data privacy and scarcity. Synthetic data generated by AI can replicate the statistical properties of real data without compromising privacy. This data can be used to train generative AI models when real data is limited or sensitive, ensuring robust model performance while adhering to privacy regulations. The ability to generate synthetic data will also facilitate the creation of diverse datasets, enhancing the robustness and adaptability of AI models.
3. Real-Time Data Processing
The ability to process data in real-time will be crucial for generative AI applications in various industries such as finance services, healthcare, etc. Advances in real-time data processing technologies will enable AI models to make instant decisions based on the latest available data. This will enhance the responsiveness and effectiveness of generative AI systems, allowing them to operate seamlessly in dynamic environments. Real-time data processing will be a key driver of innovation, allowing new applications and use cases that require immediate and accurate responses.
4. Enhanced Data Governance Practices
Data governance is set to become more stringent and evolved, driven by increasing concerns over privacy, security, and ethical use. Future data governance practices will likely include advanced techniques for ensuring data integrity, traceability, and compliance with global regulations. This will involve the implementation of blockchain technology for secure and transparent data transactions, as well as AI-driven monitoring systems to detect and prevent data breaches and misuse. Enhanced data governance will build trust in generative AI systems, promoting their wider adoption across sensitive and regulated industries.
5. Increased Focus on Data Diversity
The importance of data diversity will be increasingly recognized in the development of generative AI models. Diverse datasets will be essential to mitigate biases and ensure that AI systems are fair and inclusive. Efforts to collect and curate data from a wide range of sources and demographics will intensify, supported by community-driven initiatives and collaborations across sectors. By prioritizing data diversity, future generative AI models will be better equipped to handle a broad spectrum of scenarios and provide equitable solutions.
6. Evolution of Data-Driven Personalization
Personalization in AI-driven applications will get more improved, leveraging deeper insights from data to create highly tailored experiences. Advanced techniques such as federated learning will enable AI models to learn from decentralized data sources without compromising user privacy. This will allow for more personalized services that are both privacy-preserving and highly responsive to individual user needs. The evolution of data-driven personalization will enhance user engagement and satisfaction, making AI applications more intuitive and user-centric.
Conclusion
Throughout the blog, we understood how data plays a crucial role in generative AI. It is the foundation for training models, enhancing accuracy, ensuring diversity, driving innovation, enabling personalization, and facilitating continuous improvement. As generative AI continues to advance, the role of data will only grow more critical. To harness the capabilities of generative AI, it is crucial to seek out reliable generative AI development services, ensuring access to the expertise and resources needed to manage and utilize data effectively.
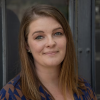
Lynn Martelli is an editor at Readability. She received her MFA in Creative Writing from Antioch University and has worked as an editor for over 10 years. Lynn has edited a wide variety of books, including fiction, non-fiction, memoirs, and more. In her free time, Lynn enjoys reading, writing, and spending time with her family and friends.