Businesses of all sizes and across all industries generate vast amounts of data on a daily basis. In order to stay competitive, organizations must find ways to transform this data into valuable insights that can inform decision-making and drive business growth. That’s where analytics comes in. Analytics is the practice of using data to identify patterns, draw conclusions, and make predictions about future events or behaviors.
There are several types of analytics, but two of the most commonly used are predictive and prescriptive analytics. In this blog post, we’ll explain what each of these approaches entails, list and describe the differences between them, and provide examples of how they can be applied to real-world business challenges.
Predictive Analytics
Predictive analytics is a branch of data analytics that uses statistical models and machine learning algorithms to analyze historical data and make predictions about future events or behaviors. The goal of predictive analytics is to identify patterns in the data that can be used to make informed decisions about the future.
How Predictive Analytics Works
To perform predictive analytics, organizations typically use sophisticated software tools that are designed to analyze large volumes of data. These tools can identify patterns and relationships in the data, and use them to generate predictions about future events or behaviors. For example, a retailer might use predictive analytics to forecast demand for a particular product, based on historical sales data, market trends, and other factors.
Examples of Predictive Analytics in Action
Predictive analytics can be applied to a wide range of business challenges. Here are a few examples:
- Fraud Detection – Banks and other financial institutions use predictive analytics to identify patterns of fraudulent behavior, such as unusual spending patterns or account access from unfamiliar locations.
- Customer Segmentation – E-commerce companies use predictive analytics to segment their customer base based on purchase history, demographics, and other factors, in order to personalize marketing campaigns and improve customer retention.
- Equipment Maintenance – Manufacturing companies use predictive analytics to predict when equipment is likely to fail, based on sensor data and other factors, so they can perform maintenance before a breakdown occurs.
Advantages and Limitations of Predictive Analytics
Predictive analytics can provide several advantages for organizations, including:
- Improved Decision-Making – Predictive analytics can provide valuable insights into future events and behaviors, which can inform decision-making across multiple areas of the business.
- Better Resource Allocation – By predicting demand for products and services, organizations can better allocate resources, reduce waste, and improve efficiency.
- Increased Revenue – Using predictive analytics to optimize pricing, promotions, and other factors enable organizations to increase revenue and profitability.
However, predictive analytics also has some limitations, including:
- Incomplete Data – Predictive analytics relies on historical data, which may not be complete or accurate, leading to inaccurate predictions.
- Over-Reliance On Algorithms – If predictive analytics software algorithms are not appropriately designed or tested, they may generate biased or inaccurate results by identifying patterns in the data.
- Lack Of Context – It can only provide insights based on the data that’s available, and may not take into account external factors that could impact future events or behaviors.
Prescriptive Analytics
Prescriptive analytics is a more advanced form of data analytics that uses historical data, predictive analytics, and other factors to make recommendations about future actions or decisions. The goal of prescriptive analytics is to provide organizations with actionable insights that they can use to optimize their operations and drive growth.
How Prescriptive Analytics Works
It requires organizations to employ prescriptive analytics tools and software that consider multiple factors when generating recommendations. These utilize algorithms and models to analyze data from various sources, such as historical data, real-time data, and external data sources and generate recommendations that help organizations optimize their operations.
With prescriptive analytics tools in place, businesses can make informed decisions that lead to improved efficiency, productivity, and profitability.
Examples of Prescriptive Analytics in Action
Prescriptive analytics can be applied to a wide range of business challenges. Here are a few examples:
- Supply Chain Optimization – Prescriptive analytics is a valuable resource for manufacturing companies seeking to enhance supply chain operations, including inventory management and transportation logistics, to reduce costs and increase efficiency. Through the use of prescriptive analytics, manufacturers can identify inefficiencies, gain valuable insights, and make informed decisions to streamline operations and maximize profitability.
- Marketing Campaign Optimization – Retailers and e-commerce companies utilize this to optimize their marketing campaigns, by identifying the most effective channels, messages, and offers for different segments of their customer base.
- Customer Experience Optimization – Service-based businesses use prescriptive analytics to optimize the customer experience, by identifying areas of the business that are most likely to impact customer satisfaction, such as call center interactions, website usability, and product quality.
Advantages and Limitations of Prescriptive Analytics
Prescriptive analytics can provide several advantages for organizations, including:
- Actionable Insights – Prescriptive analytics provides organizations with specific recommendations that they can use to optimize their operations and drive growth.
- Real-Time Decision-Making – Organizations can make prompt decisions and take swift action using prescriptive analytics software, which is capable of analyzing real-time data. This capability empowers businesses to respond quickly to market changes and make data-driven decisions, resulting in improved efficiency and productivity.
- Competitive Advantage – By using prescriptive analytics to optimize their operations, organizations can gain a competitive advantage over rivals who rely on less sophisticated analytics tools.
Limitations
- High Cost – Prescriptive analytics tools and software can be expensive to implement and maintain, making them less accessible to small and medium-sized businesses.
- Complexity – This requires a high degree of technical expertise and specialized skills, which can make it difficult for organizations to fully leverage the power of prescriptive analytics.
- Ethical Considerations – It can raise ethical concerns, such as privacy and data security if organizations are not careful about how they collect, use, and store data.
Differences Between Predictive and Prescriptive Analytics
While predictive and prescriptive analytics are both valuable tools for organizations, there are several key differences between the two approaches:
Goal Of Each Approach
The goal of predictive analytics is to make predictions about future events or behaviors, while the goal of prescriptive analytics is to make recommendations about future actions or decisions.
Types Of Data Used
Predictive analytics typically relies on historical data, while prescriptive analytics can incorporate real-time data, external data sources, and other types of data.
Analytical Techniques Used
Predictive analytics uses statistical models and machine learning algorithms to analyze data and generate predictions, while prescriptive analytics uses optimization algorithms and decision-making models to generate recommendations.
Business Applications
Predictive analytics is commonly used for applications such as fraud detection, customer segmentation, and equipment maintenance. Prescriptive analytics is commonly used for applications such as supply chain optimization, marketing campaign optimization, and customer experience optimization.
Use Cases for Prescriptive and Predictive Analytics
Predictive and prescriptive analytics can be applied to a wide range of business challenges. Here are some specific use cases for each approach:
Predictive Analytics Use Cases
- Fraud detection
- Customer segmentation
- Equipment maintenance
- Sales forecasting
- Market trend analysis
Prescriptive Analytics Use Cases
- Supply chain optimization
- Marketing campaign optimization
- Customer experience optimization
- Pricing optimization
- Product development optimization
Which Approach is Right for Your Business?
When deciding whether to use predictive or prescriptive analytics, organizations should consider factors such as the complexity of their business operations, the availability of data, the cost of implementing and maintaining analytics tools, and the skills and expertise of their analytics team. In some cases, a combination of predictive and prescriptive analytics may be the best approach.
Predictive and prescriptive analytics are powerful tools that can help organizations turn data into valuable insights and drive business growth. While predictive analytics is focused on making predictions about future events or behaviors, prescriptive analytics takes things a step further by providing specific recommendations for future actions or decisions. Both approaches have their advantages and limitations, and organizations should carefully consider their business needs and the availability of data and resources before deciding which approach to use. By leveraging the power of predictive and prescriptive analytics, organizations can gain a competitive edge, optimize their operations, and drive growth in an increasingly data-driven world.
In addition, it’s worth noting that the field of analytics is rapidly evolving, and new tools and techniques are emerging all the time. To stay ahead of the curve, organizations should invest in ongoing training and development for their analytics teams, and be willing to experiment with new approaches and technologies as they become available. With the right mindset, tools, and expertise, any organization can harness the power of analytics to unlock valuable insights and drive business success.
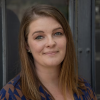
Lynn Martelli is an editor at Readability. She received her MFA in Creative Writing from Antioch University and has worked as an editor for over 10 years. Lynn has edited a wide variety of books, including fiction, non-fiction, memoirs, and more. In her free time, Lynn enjoys reading, writing, and spending time with her family and friends.